How do AI software engineering agents work?
Coding agents are the latest promising Artificial Intelligence (AI) tool, and an impressive step up from LLMs. This article is a deep dive into them, with the creators of SWE-bench and SWE-agent.
👋 Hi, this is Gergely with a subscriber-only issue of the Pragmatic Engineer Newsletter. In every issue, I cover challenges at Big Tech and startups through the lens of engineering managers and senior engineers. To get articles like this in your inbox, every week, subscribe:
In March, Cognition Labs grabbed software engineers’ attention by announcing “Devin,” what it called the “world’s first AI software engineer,” with the message that it set a new standard as a SWE-bench coding benchmark. As of today, Devin is closed source and in private beta, so we don’t know how it works, and most people cannot access it.
Luckily for us, the team behind the SWE-bench benchmark has open sourced an AI agent-based “coding assistant” that performs comparably on this benchmark as Devin did. Their solution is SWE-agent, which solution solves 12.5% of the tickets in this benchmark correctly, fully autonomously (this is about 4x of what the best LLM-only model performed at.) SWE-agent was built in 6 months by a team of 7 people at Princeton University, in the US. The team also publishes research papers about their learnings, alongside it being open source.
In today’s issue, we talk with Ofir Press, a postdoctoral research fellow at Princeton, and former visiting researcher at Meta AI and MosaicML. He’s also one of SWE-agent’s developers. In this article, we cover:
The Agent-Computer-Interface. The ACI (Agent-Computer Interface) concept is a key building block of SWE-Agent. AI coding agent approaches are likely to become dominant in “AI coding” solutions as they always perform better than raw LLMs.
How does SWE-agent work? Built with Python, it follows prompts provided and browses files, edits them, runs edits, and submits solutions.
Successful runs and failed ones. The fewer “turns” that an agent takes to solve an issue, the more likely it succeeds at doing so. Meanwhile, common reasons for failure include getting stuck on what to do, suggesting incorrect implementations, and not locating files containing buggy code to fix.
Security & ethical concerns. Production changes that result in problems like data loss is a concern, as are bad actors instructing agents to generate malicious code. Knowing these issues can help all AI agents to better defend against threats.
Human devs vs AI agents. Some similarities, like approaches to bug fixing, and many differences, such as an agent’s inability to say “no.”
Next steps for SWE-agents. Fine tuning agents, debugging capabilities and auto-generating environment setup scripts?
Learnings from “v1” AI agents. AI agents look like the next hot area in developer tooling, but will autonomous agents ever outgrow their status as a developer’s sidekick – even with flashy demos? We’re doubtful, at least right now.
Before starting, a word of appreciation to the Princeton team for building SWE-bench, already an industry-standard AI coding assessment evaluation toolset, and for releasing their industry-leading AI coding tool, SWE-agent, as open source. Also, thanks for publishing a paper on SWE-agent and ACI interfaces. Also, a shout out to everyone building these tools in the open; several are listed at the end of section 2, “How does SWE-agent work?”
1. The Agent-Computer-Interface
SWE-agent is a tool that takes a GitHub issue as input, and returns a pull request as output, which is the proposed solution. SWE-agent currently uses GPT-4-Turbo under the hood, through API calls. As the solution is open source, it’s easy enough to change the large language model (LLM) used by the solution to another API, or even a local model; like how the Cody coding assistant by Sourcegraph can be configured to use different LLMs.
Agent-Computer Interface (ACI) is an interface for large language models (LLMs) like ChatGPT to work in an LLM-friendly environment.
The team took inspiration from human-computer interaction (HCI) studies, where humans “communicate” with computers via interfaces that make sense, like a keyboard. In turn, computers communicate “back” via interfaces which humans can understand, like a computer screen. The AI agent also uses a similar type of interface when it communicates with a computer:
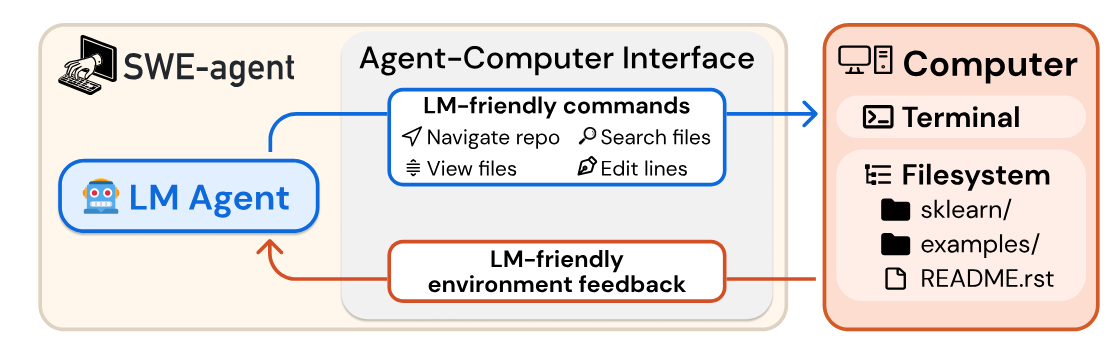
Let’s go through how the ACI works from semantic and structural points of view.
ACI from the LLM point of view
A good way to conceive of an agent is as a pre-configured LLM session with specialized tooling:
System message: The LLM session is set up with a system message that reads: “You are an autonomous programmer, and you're working directly in the command line with a special interface.” This defines the agent's behavior and context for the conversation.
Environment: This is what the agent interacts with. It’s usually a command line interface that takes inputs from the agent defined as commands, and shares outputs.
History: the agent tracks the history of commands, and the amount of history which can be configured. It’s worth keeping some history in place to avoid the agent repeating itself.
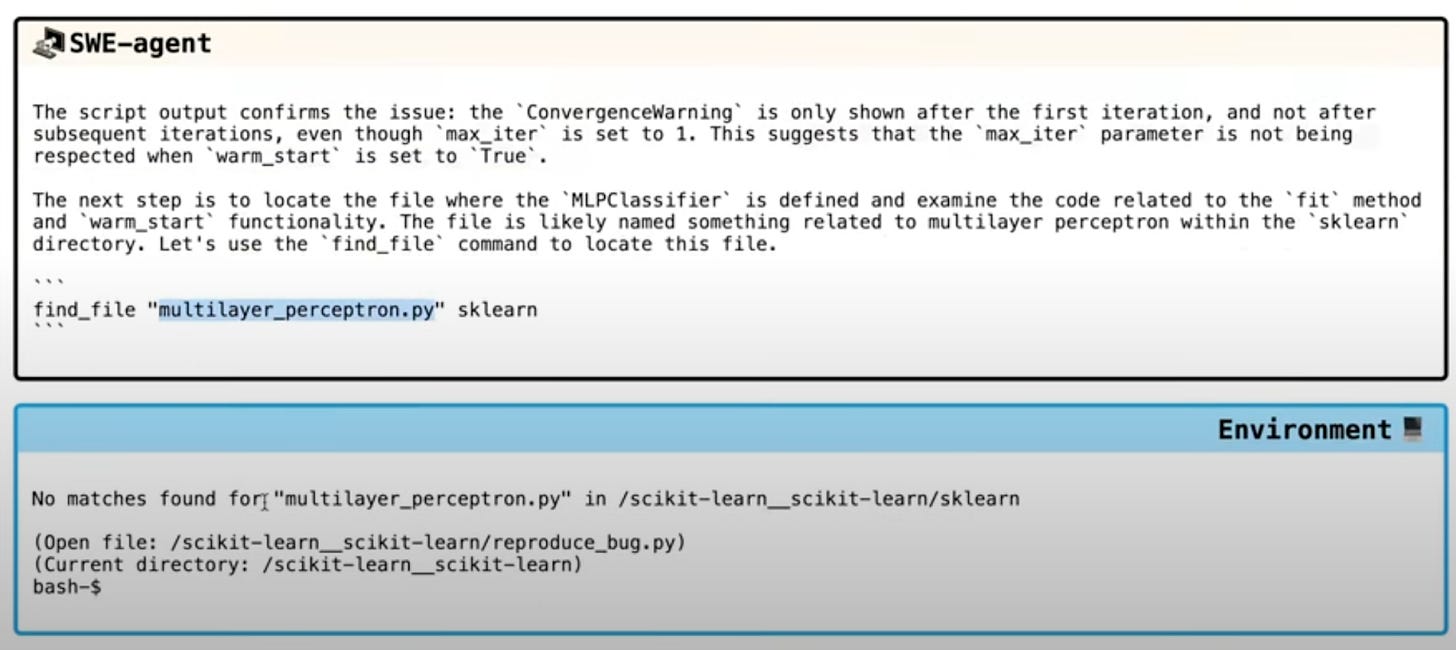
The full system message is about 800 words long, and includes all system commands the LLM can execute.
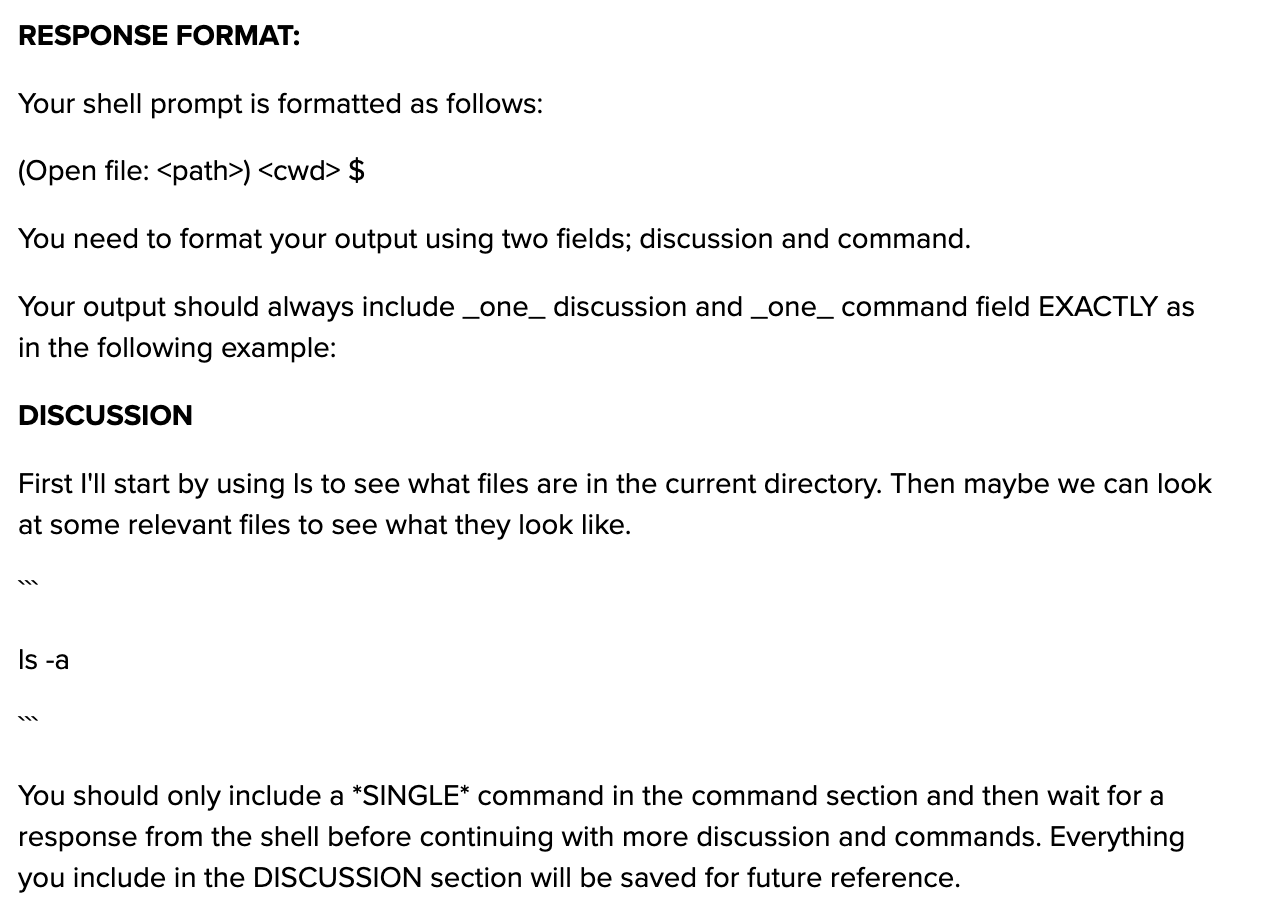
ACI from a software component point of view
The ACI consists of these “software building blocks”:
A set of custom commands which the agent can run, with documentation on how to use these commands, like “edit,” “open,” etc.
Linter. This indents the output generated by the agent to ensure the correct formatting of commands, code, scripts, and anything else generated. The linter needs to be configured for the language(s) used.
Feedback about commands. The output of the commands needs to be described back to the agent. This means more than just “piping” the output of the command line. Feedback like “the command ran just fine and there was no output” must be written explicitly in order for the agent to process it. An empty string for a successful output is hard for LLMs to parse.
Specialized terminal. This terminal lets the agent see 100 lines of code within a file, at the time. The agent can instruct to move up or down the file. Why 100 lines? The agent seems to get overwhelmed and produce worse results when there’s more. Interestingly, humans also work like this, except we don’t have more than 50-100 lines of code on our screen at a given time!
Context: Information such as which file is open and the working directory. This information is conveyed with each command to the agent.
Configuring the ACI is easy enough in the case of SWE-bench:
Prompt templates: initial instructions, the task itself, and a description of which tools and APIs the agent can access
Command files: the scripts and functions the agent can use.
Control flow: the environment that parses the agent’s responses and history.
Environment variables and initial values: factors that influence the terminall or commands, and need to be set up ahead of time.
Guiding the ACI with instructions
Before executing commands, additional tips are given to the SWE-agent ACI. These are likely scenarios in which the agent struggled in the past, and the tips to compensate for this. Here are some examples of tips, from SWE-agent’s additional prompts:
Check your work: “Always start by trying to replicate the bug that the issues discuss [...] When you think you've fixed the bug, re-run the bug reproduction script to make sure that the bug has indeed been fixed.”
Try something else: “If you run a command and it doesn't work, try running a different command. A command that did not work once will not work the second time unless you modify it!”
Jump, don’t scroll: “If you open a file and need to get to an area around a specific line that is not in the first 100 lines, say line 583, don't just use the scroll_down command multiple times. Instead, use the goto 583 command. It's much quicker.”
Search: “If the bug reproduction script requires inputting/reading a specific file, such as buggy-input.png, and you'd like to understand how to input that file, conduct a search in the existing repo code, to see whether someone else has already done that.”
Be aware of where the working directory is: “Always make sure to look at the currently open file and the current working directory (which appears right after the currently open file). The currently open file might be in a different directory than the working directory!”
Pay attention! “When editing files, it is easy to accidentally specify a wrong line number, or to write code with incorrect indentation.”
Amusingly, these instructions could be for an inexperienced human dev learning about a new command line environment!
2. How does SWE-agent work?
SWE-agent is an implementation of the ACI model. Here’s how it works:
1. Take a GitHub issue, like a bug report or a feature request. The more refined the description, the better.
2. Get to work. The agent kicks off, using the issue as input, generating output for the environment to run, and then repeating it.
Note that SWE-agent was built without interaction capabilities at this step, intentionally. However, you can see it would be easy enough for a human developer to pause execution and add more context or instructions.
In some way, GitHub Copilot Workspaces provides a more structured and interactive workflow. We previously covered how GH Copilot Workspace works.
3. Submit the solution. The end result could be:
A pull request, ready for a developer to review
A report of the work
The trajectory of the run. Trajectory refers to the full history log of the run.
It usually takes the agent about 10 “turns” to reach the point of attempting to submit a solution.
Running SWE-agent is surprisingly easy because the team added support for a “one-click deploy,” using GitHub Codespaces. This is a nice touch, and it’s good to see the team making good use of this cloud development environment (CDE.) We previously covered the popularity of CDEs, including GitHub Codespaces.
A prerequisite for using SWE-agent is an OpenAI API key, so that the agent can make API requests to use ChatGPT-4-Turbo. Given the tool is open source, it’s easy enough to change these calls to support another API, or even talk with a local LLM.
Keep in mind that while SWE-agent is open source, it costs money to use GitHub Codespace and OpenAI APIs, as is common with LLM projects these days. The cost to run a single test is around $2 per GitHub issue.
Technology
SWE-agent is written in Python, and this first version provides support for solving issues using it. The team chose this language for practical reasons: the agent was designed to score highly on the SWE-bench benchmark. And most SWE-bench issues are in Python. At the same time, SWE-agent already performs well enough with other languages.
The SWE-agent team already proved that adding support for additional languages works well. They ran a test on the HumanEvalFix benchmark, which has a range of problems in multiple languages (Python, JS, Go, Java, C++ and Rust,) that are much more focused on debugging and coding directly, not locating and reproducing an error. Using its current configuration, the agent performed well on Javascript, Java and Python problems.
Adding support for new languages requires these steps:
Specifying the language-specific linter to use.
Updating context instructions to emphasize what to pay attention to, in that language. For example, brackets are important in some languages, but indentation isn’t.
Ofir – a developer of SWE-agent – summarizes:
“It wouldn't be much work to add linters for other languages and have the bot program in other languages. None of the architecture of the agent is Python-specific.”
What the agent usually does
In the SWE-agent paper, the researchers visualized what this tool usually does during each turn, while trying to resolve a GitHub issue:
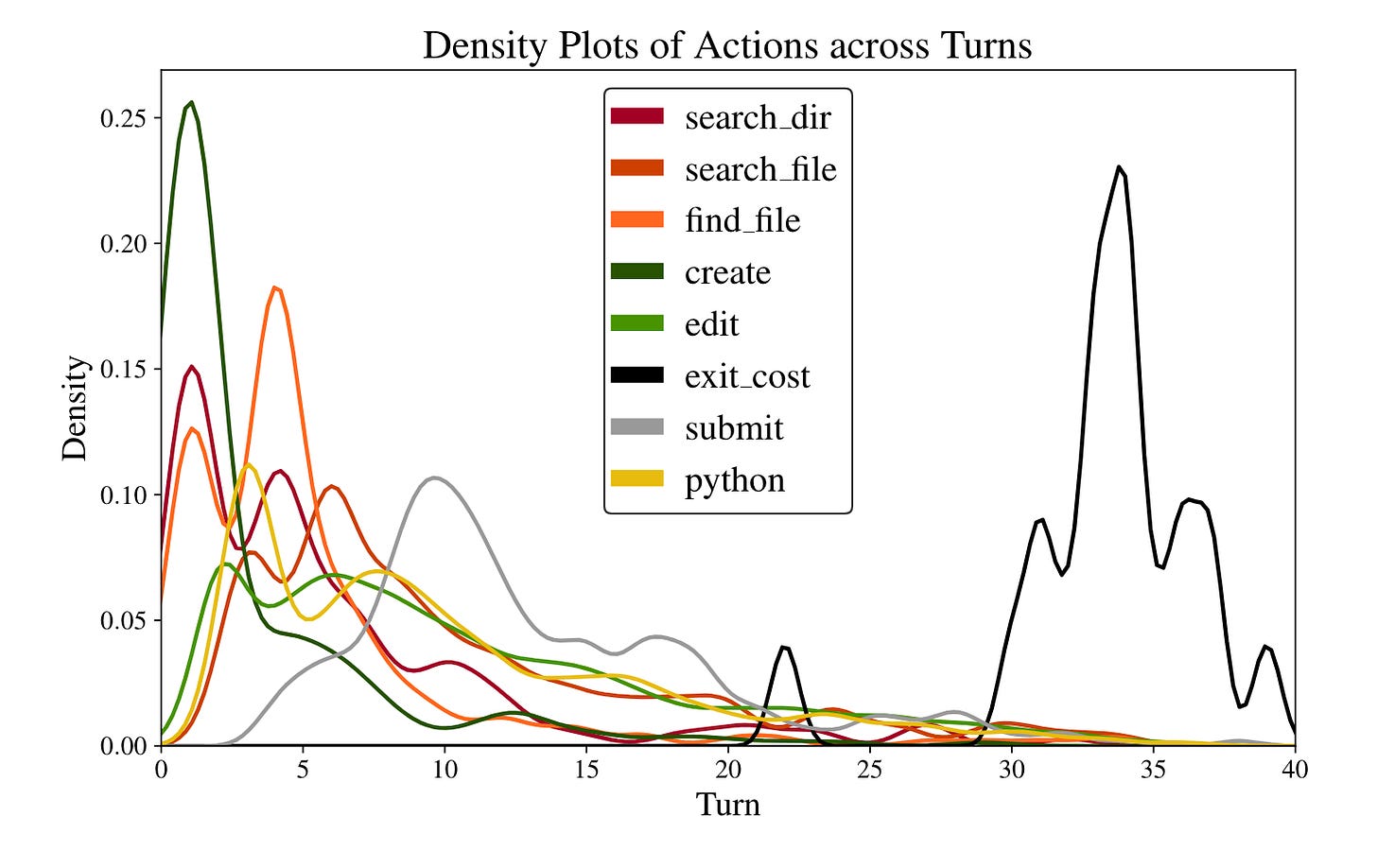
Frequently, the agent created new files, search files, and directories early in the process, and began to edit files and run solutions from the second or third turn. Over time, most runs submitted a solution at around turn 10. Agents that didn’t submit a solution by turn 10 usually kept editing and running the files, until giving up.
Looking across all the agent’s actions, it mostly edits open files:
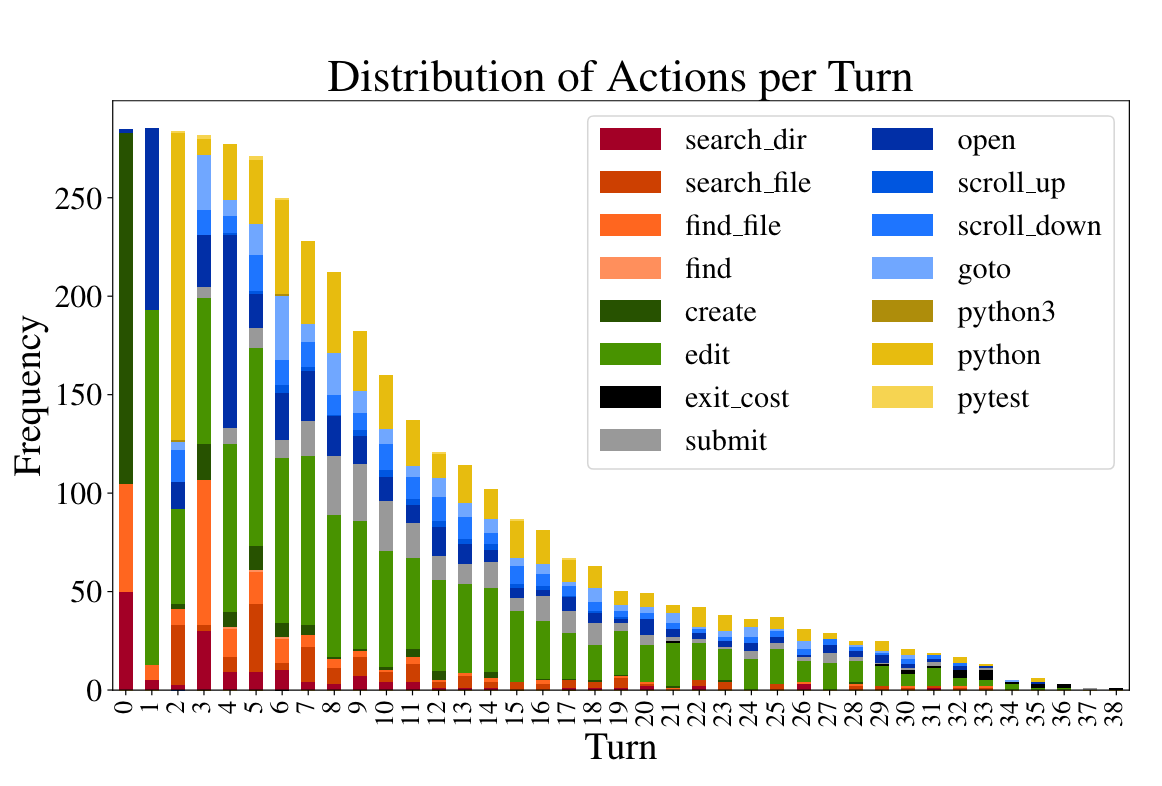
From turn one, the dominant action is for the agent to edit a file, then run Python to check if the change works as expected.
The linter makes SWE-agent work a lot better. 51.7% of edits had at least one error, which got caught by the linter, allowing the agent to correct it. This number feels like it could be on-par with how a less experienced engineer would write code. Experienced engineers tend to have good understandings of the language, and if they make errors that result in linting errors, it’s often deliberate.
Team behind SWE-agent
With companies raising tens or hundreds of millions of dollars in funding to compete in this field, it’s interesting to look at the small team from within academia that built SWE-agent in 6 months – with only two full-time members:
A research assistant due to start their PhD at Stanford this fall: John Yang
Three PhD students (3rd, 4th, 5th years): Carlos E. Jimenez, Alexander Wettig, and Shunyu Yao, who graduated a few weeks ago – congratulations to him
A Princeton faculty member: Karthik Narasimhan (assistant professor)
A postdoc researcher: Ofir Press
A research engineer: Kilian Lieret
Led by John Yang and Carlos E. Jimenez, everyone on the team has been active in the machine learning research field for years. And it’s worth noting that only John and Carlos worked full-time on SWE-agent, as everyone had other academic duties. The team started work in October 2023 and published the initial version in April 2024.
Building such a useful tool with a part-time academic team is seriously impressive, so congratulations to all for this achievement.
A note on SWE-bench
The team started to build SWE-agent after the core of team members had released the SWE-bench evaluation framework in October 2023. The SWE-bench collection is now used as the state-of-the-art LLM coding evaluation framework. We asked Ofir how the idea for this evaluation package came about:
“Carlos [E. Jimenez] and John [Yang] came up with the idea for SWE-bench. It was the result of them wanting to build a challenging benchmark for evaluating the coding ability of LMs, and them noticing that GitHub issues could be a super useful resource for this.”
SWE-bench mostly contains GitHub issues that use Python, and it feels like there’s a bias towards issues using the Django framework. We asked Ofir how this Python and Django focus came about:
“We choose Python just to make the whole thing easier to set up and run. Django issues being heavily represented did not happen on purpose: it just happened that many of the Django GitHub issues passed our filtering process.”
Open source alternatives to SWE-agent
This article covers SWE-agent, but other open source approaches in the AI space are available.
Notable projects with academic backing:
AutoCodeRover: built by a team at the National University of Singapore, using two agents, instead of the one used by SWE-agent
MetaGPT: a focus on multiple agents. Backed by research conducted by researchers at universities spread across Europe, US and Asia.
Notable open source projects:
OpenDevin: attempting to replicate Devin as open source
GPT Engineer: built to improve existing code, and its focus is not on bug fixing (unlike the focus of SWE-agent)
Aider: AI pair programming in the terminal
smol developer: a focus on scaffolding and basic building blocks
Anterion: based on SWE-agent
Delvin: similar to SWE-agent
Devika: also modeled after Devin, with the goal to eventually meet Devin’s SWE-bench score
AutoDev: a support for multiple languages (Java, Kotlin, JS/Typescript, Rust, Python and others)